How analytics is moving intelligence to the edge
Tags
The proliferation of intelligent devices has skyrocketed. The price of performant, low-powered, cheap microprocessors has driven the development of intelligent devices – smart doorbells, smart energy meters, smart watches, and more. The availability of ubiquitous networks including 5G and Low Power Wide Area Networks (LoRaWAN) deliver data to and from devices with certainty.
A range of vendors now offer fully formed suites of services using the Internet of Things (IoT) methodology. These three core elements have contributed to an explosion in the number of connected devices – 14.4 billion in 2022, rising by 18 percent in 12 months.
With this comes the need to manage vast amounts of data, ensure devices are backed-up, and that they communicate effectively with other intelligent systems – be they in the cloud or locally. How can that be achieved?
The challenge: managing disparate data, and lots of it
We already use billions of devices that generate and share data with other devices and systems in the cloud. Think of an internet home hub connected to a series of smart locks, cameras, and sensors – a network of smart, low powered devices that can connect to a remote gateway, share data, and then direct data onwards to a central service in the cloud.
But what happens when there is no network coverage, or the volume of data is so high that the cost of connecting a device becomes prohibitive? As most services charge by the volume of information transmitted, businesses offering such solutions need to be cognisant of data volumes to ensure the service is cost-effective and affordable for mass consumption.
Take the smart heating system. Typically, they are driven by an electronic thermostat. We set the desired temperature, and the time that the heating comes on and off. Many systems are now combined with a smart meter, conveying consumption figures back to the energy supplier and negating the need to read the meter.
Early systems measured house temperature either from one source – the thermostat – or multiple complimentary sensors, relaying similar temperature readings every 30 minutes. This huge amount of data burdened consumers’ broadband accounts as well as energy suppliers’ cloud capacity. The result? System-wide cost. Then, in this scenario, factor in a break in broadband connectivity. What happens to the data then? How do we ensure a continuity of service?
The answer: developing feature-rich operating systems
Over the last five years there has been a steady development of lightweight, real-time operating systems that turn intelligent devices into their own minicomputers. This development has been taken further to create feature-rich operating systems that support remote applications and services, transforming remote devices into potential gateways with their own network – a system of systems. These services can make decisions on the gateway, or at the ‘edge’, removing some of the need to transport data back to the cloud. The benefits include saving on the transmission of data to the cloud, reducing bandwidth usage, and potential cost. Additionally, edge systems continue to operate even when the wider network is down, providing continuity of service to the user.
A good example is Amazon Web Services’ (AWS) IoT Greengrass Operating System, supported by their analytics service, SageMaker. Greengrass is an open-source edge runtime and cloud service for building, deploying, and managing device software, and is typically deployed in smart devices that are used as gateways to manage remote devices with limited micro-processer capabilities, like simple sensors.
Engineers can develop and train Machine Learning (ML) models in the cloud using SageMaker, test them, and then deploy them on Greengrass as ‘ML Inference’. Think of it as a lightweight version of the model built in the cloud that can live in the gateway device, performing decision-making commands about the data presented. This Greengrass configuration can continue to work even if the connectivity back to the cloud is broken, resuming connection when the network returns.
Smart devices are becoming smarter
To show the benefits of AWS Greengrass in action, let’s look at the heating system example. A smart heating controller that contains AWS Greengrass will monitor the house temperature. Two analytics services, built using SageMaker models, are deployed onto Greengrass, one to detect changes in temperature based on a four-degree swing, and the other to detect a steady decline of ten degrees over a five-hour period. If either of these conditions are detected, a data packet will be sent to the cloud service alerting the supplier of a fundamental change.
The only data transmitted may be a single daily average temperature reading, plus temperature swings of four-degrees – indicating a boiler cut out, or a ten-degree drop – suggesting a possible heating system failure. The energy supplier can then agree that if either state is induced, an SMS text will be sent to the homeowner to alert them. If the temperature swing was planned, the homeowner can ignore the alert, but if not, they can arrange an engineer call-out or remotely adjust their heating system. The result is that the smart devices become smarter, offering a richer system with more economic data usage and therefore lower cloud charges – all accelerated by analytics deployed at the edge.
If you abstract the same thinking into other problems and markets, such as managing systems onboard trucks and trains, or multiple managements systems that operate an office block, you can start to see how analytics performing machine learning at the edge will be a game-changer for IoT. It can supercharge the capability of the billions of devices within our increasingly digital lives.
Explore more
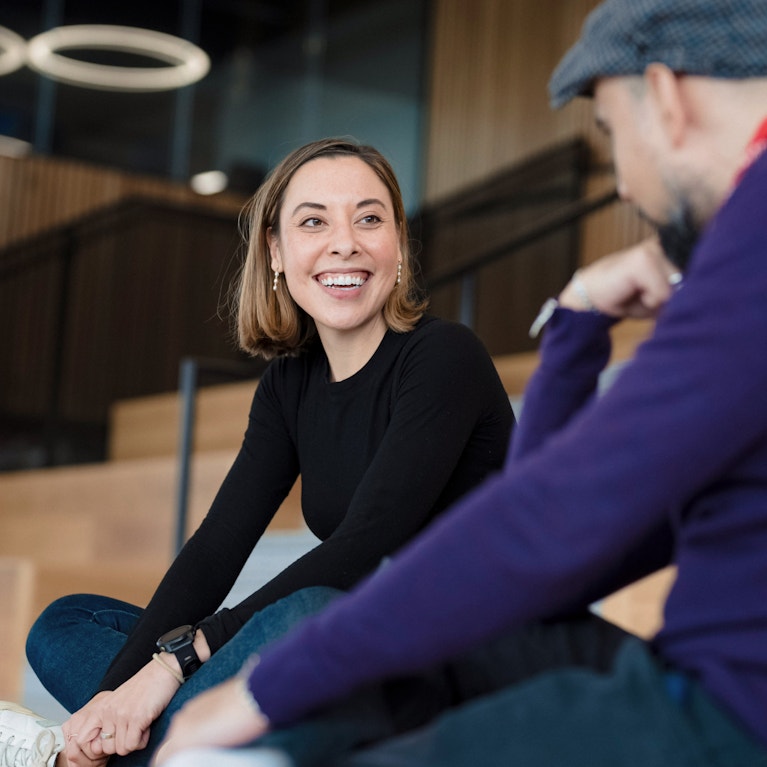