Unlocking population-wide clinical trial insights through health care data
Tags
The ability to design clinical trials that deliver quicker results, and are more adaptive to changing environmental conditions, is a panacea for many sponsors and contract research organisations (CROs). Increasingly, focus is being placed on how to use patient segmentation to ensure appropriate trial design, site selection, and recruitment approaches.
To make the best possible decisions, organisations involved in clinical research require access to large-scale and relevant, data sets with the appropriate legal and ethical consents.
Historically the route to acquiring these datasets has either been through partnership or acquisition, such as 23andMe’s collaboration with GSK. Recent studies on both sides of the Atlantic show an increasing willingness from members of the public to share their data to aid in the development of new treatments. More interestingly, people are also comfortable with their data being used across multiple studies. Members of the public directly sharing their health data with clinical research organisations is not unexplored, but the level of willingness highlighted in these studies is much higher than many would expect. The size of the potential data sets that could be generated would transform the way that researchers could use longitudinal analysis to derive valuable cross-population and cross-trial insights. This opens the question of how organisations can look to implement these approaches.
Ensuring data security
No approach will be successful without establishing and maintaining trust with the individuals who are sharing their information. To ensure the safety and security of this sensitive data, global organisations should look to the examples set within the EU and apply the stringent privacy practices to enable the reuse of anonymised clinical data for other purposes.
Organisations must be clear with potential data donors on how the data will be used and ensure that there are appropriate controls in place that dictate how the information can be queried by research and clinical operations teams. There are many examples of how to approach this established by large national and international organisations, such as how NHS England defines its suppression rules to avoid the unintended disclosure of information about small groups of individuals (typically those below ten and 25 individuals).
Ever since the introduction of HIPAA was sparked by the identification of Governor William Weld’s medical history from within an insufficiently managed data set, the importance of proper anonymisation of complex health data sets has been understood. Maintaining individuals’ anonymity across significant volumes of interconnected anonymised and pseudonymised data sets will be challenging. The approach taken by initiatives such as the UK’s Secure Data Environments should be examined by other organisations who are looking to take steps down this path.
Scalable data management
The nature of medical treatment means that, even with the widespread use of Electronic Patient Records, the vast majority of healthcare data is unstructured and scattered across multiple different formats – a challenge which is only increasing with the rise of data livestreaming through digitally enabled medical devices. The fast-paced nature of front-line health care and diversity of systems (many of which are significantly out of date) often means that the quality levels of this data are far below what is typically seen in clinical research databases.
This provides a highly promising use case for AI-driven Natural Language Processing (NLP). NLP can help to extract meaningful insights from the unstructured data and work across various system and file formats to align equivalent data which is held in multiple different ways. This significantly reduces time and cost that organisations would otherwise incur in the creation of comprehensive data sets.
Once these data integration mechanisms are in place it opens the possibility for alignment of other data sources, such as data captured through in silico trials. By broadening the breadth and depth of data that clinical research organisations have access to, we can open the way to identifying and engaging with previously unknown patient populations.
The use of public health data does not have to be limited to patient segmentation activities. Overlaying AI-driven analysis tools onto this information allows clinical operations teams to retrospectively assess patient health data to uncover potential areas of therapeutic enquiry that would previously have been hidden. The insights gathered by John Hopkins University researchers on the use of AI to identify patients at risk of a heart attack, demonstrates how impactful the use of data which is normally overlooked can be.
Evaluation can also extend post-licencing, with organisations moving beyond patient or HCP-led side effect reporting (like MHRA’s yellow card scheme) into the live evaluation of prescriptions, diagnosis, and treatment data to understand the ongoing risk profile of their drug in highly specific patient populations. Although primarily targeted at operational challenges, The American College of Emergency Physicians’ EM Data Institute is an example of how national data sets can be leveraged to help increase the safety of drugs already on the market and to support the identification of new clinical targets.
Regulatory impacts
There is rarely deep and meaningful uptake of new technologies or approaches without clear direction from regulatory bodies. Luckily for those who are interested in engaging proactively with the public to begin the build of health data sets, the FDA has already initiated a discussion paper that touches on the use of AI to assess real-world data whilst the EMA has a significant effort through its HMA-EMA Big Data Task Force.
It is interesting to consider some of the longer-term regulatory implications that these approaches may bring. As the analysis of large sets of linked data, significant volumes of which may be self-reported, increases, regulatory authorities will need to be stricter on ensuring that submissions are appropriately considering the necessary statistical controls to prevent the multiple comparison problem from arising – an issue that those who work in genomics are all too aware of. Additionally, an increased ability to reinterrogate the formative clinical trials that drove a drugs development may allow agencies to more frequently require retrospective analysis if unexpected side effects or altered efficacy are identified in specific patient cohorts.
Key takeaways
We see two key steps that organisations must take to fully embrace the potential of widespread public involvement in data sharing for clinical trials:
Establish trust and embrace transparency
Earning the trust of the public and ensuring that your organisation continues to operate in a manner that will maintain this trust, is imperative. These approaches must be aligned with action and organisations must revisit data protection and security policies to enable the safe use of emerging technology in clinical research, including ethical AI, privacy, and cyber considerations.
Organisations should also look to examples in other industries where this has been done successfully. Key hallmarks of organisations which members of the public continually engage with data sharing are ones in which individuals can maintain complete control over information they are sharing and have a clear line of sight to the value that they are deriving, or in the case of clinical trials, providing. The UK’s National Institute for Health Research is a pioneer in this space, with its Be Part of Research initiative offering a convenient and streamlined front door for members of the public to take part in clinical research.
Loosen the bindings of legacy tech
Moving away from the historical systems is no easy task, especially within the clinical trial environment. Change must be championed internally to establish a clear strategic direction for the organisation, reaching across both technical teams and all clinical functions that can derive powerful insights from these wide-reaching capabilities.
Organisations must establish the technical infrastructure needed to capture, process and connect relevant data across disparate internal systems, and establish the right tools and processes to ingest, transform, analyse, and action the insights that arise.
Research programmes such as Our Future Health or long-running registries such as the UK Biobank show how members of the public will willingly share their health data with trusted organisations to support clinical research and reiterating the significance of altruism as a key driver behind an individual’s willingness to take part in a clinical trial. These historical examples can act a model for organisations who wish to understand how to successfully build and maintain trust with the public, protect participants data, and establish mechanisms for collaborating with partners in a much wider external ecosystem.
This article was originally published in International Clinical Trials (ICT).
Explore more
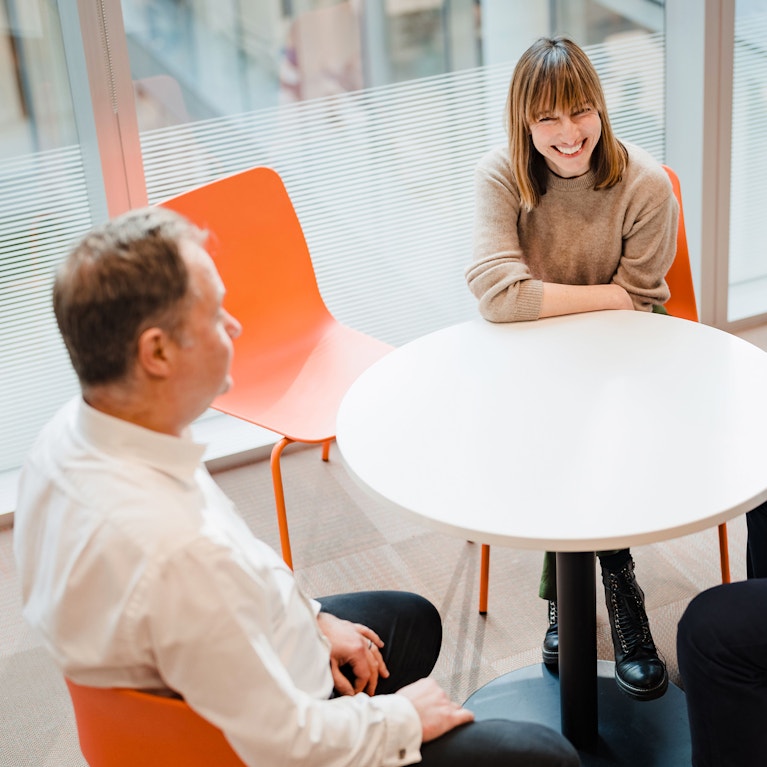