Predictive analytics: How utilities are moving from reactive to proactive
Tags
Americans need reliable electricity more than ever as they work from home and charge electric vehicles in greater numbers. But in 2020, the average U.S. electrical utility customer experienced more than eight hours of power disruptions — the highest amount on record since the U.S. Energy Information Administration began keeping track in 2013. Wildfires, severe weather, and climate change are wreaking havoc on utilities’ ability to keep the lights on. Even when hurricanes and natural disasters are removed from the equation, the average utility customer experienced slightly more outage time in 2020 than in 2013.
Yet utilities aren’t powerless in the fight to increase reliability and resiliency. Predictive analytics are unlocking a wealth of data utilities already have, allowing them to improve service for their customers.
While distribution system power quality (PQ) data has long been available, it has traditionally been used in a limited manner, such as looking at why voltage was fluctuating on a system. Today, utilities are using predictive analytics that leverage PQ data and smart devices that already exist to be more proactive and predict equipment failure.
When integrated with supervisory control and data acquisition (SCADA) and other connectivity models, predictive analytics tools are predicting the failure of an asset and its approximate location — a boon for utilities trying to increase reliability, resiliency, and agility to better serve their customers.
Connectivity is key for predictive analytics
Advanced predictive analytics systems act as a “universal translator” of device communications, making previously siloed data useful for predicting equipment failures. Connecting disparate systems so they can communicate has proved a huge barrier to enabling effective predictive analytics solutions in the past.
But advances in technology have made it easier for systems to begin “talking,” so data can be aggregated and become truly “critical/key data” to enable advanced analytics. Machine learning algorithms using this data are finding nuances in the grid that might have not been so evident before, and utilities can be notified to replace devices before they fail.
Use of predictive analytics in the utility industry is still in its infancy, though some utilities like Florida Power & Light Company (FPL) have used it for quite a while. In 2007, FPL began deploying smart meter technology, which opened the door for analytics. Fifteen years later, the investment has proven its worth by making FPL far more efficient. Customers have benefited, with day-to-day reliability improving 40% since 2007.
For example, FPL eliminates thousands of unnecessary truck rolls every year by using analytics from smart meters. The better information allows FPL to more efficiently target resources, helping speed restoration for customers.
Predictive analytics on underground power lines
Predictive capability essentially turns traditional asset management on its head because utilities no longer need to rely solely on periodic inspections.
A classic example is underground power lines with failing insulation emitting partial discharges and then subsiding in a predictable pattern until the insulation finally fails. Today, FPL has been able to shift some unplanned outage restoration work to planned maintenance by predicting and addressing failures on thousands of customer service lines as well as hundreds of transformers before those failures become outages.
FPL is actively expanding this capability by developing a model to predict underground power line failures using high-frequency sampling data. These advances can eliminate outages for customers or minimize their impact by allowing customers to prepare for a scheduled outage. The cycle of prediction, prevention, fewer outages, and improved ability to do productive work has helped FPL become more forward-looking, agile, and less reactive.
The “virtuous cycle” created by using predictive analytics has allowed FPL to become a more effective utility that provides better reliability for its customers.
Should an outage occur, FPL knows the moment a customer loses power and can immediately dispatch the closest restoration specialist, all without a call from the customer. Quicker and easier power restorations — and fewer restorations overall — benefit the utility and its customers.
Predicting the future of predictive analytics
The future of predictive analytics for utilities appears bright, as companies pursue goals of identifying and integrating critical data, developing more mature analytics, meeting future energy demands, and reducing customer outages.
FPL continues to identify and discover new use cases with the insights that predictive analytics offer, including knowing when a line switch or capacitor bank isn’t performing correctly. The utility envisions a future where it can locate a problem within 50 feet, allowing for quicker resolution and less customer impact.
As the use of predictive analytics in the industry matures, the relationship between green energy, electrification, resilience, reliability, and more sophisticated algorithms should make the use case for the technology even more compelling. As more utilities unlock the capabilities of predictive analytics, customers should benefit from service that is more efficient and reliable than ever before.
This article was first published in Powergrid International.
Explore more
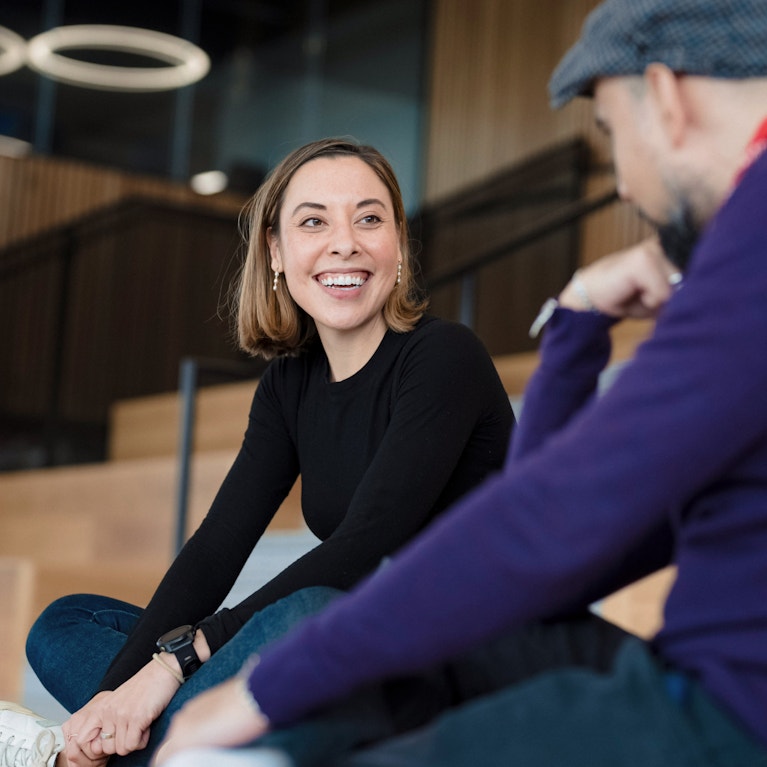